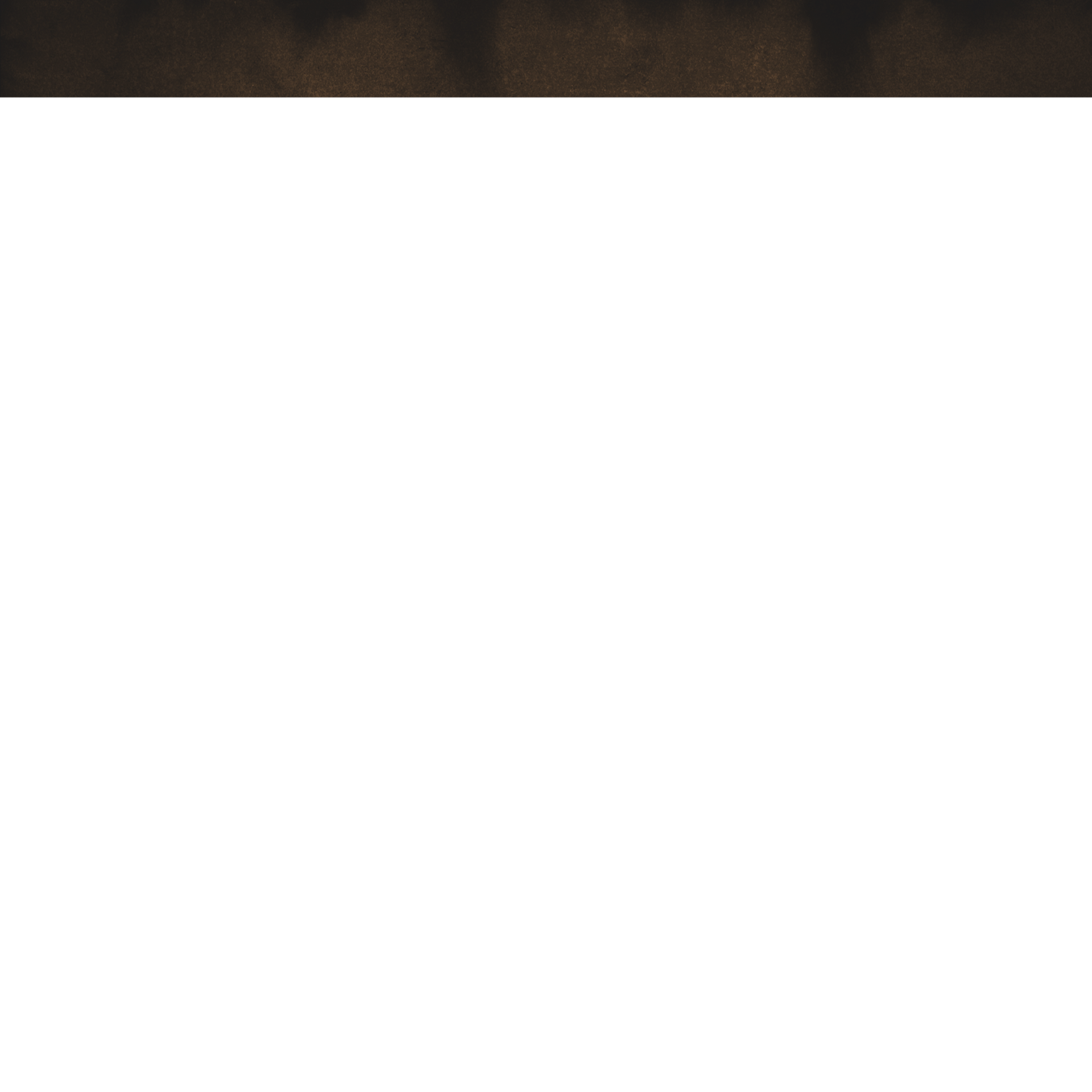
Learning Site-specific Probing Beams for Fast mmWave Beam Alignment
Dec 2020–Dec 2021, UT Austin WNCG
Dr. Jianhua Mo, Prof. Jeffrey G. Andrews
I proposed a beam alignment method for millimeter-wave (mmWave) systems that uses the beam sweeping measurements of a small site-specific probing codebook to predict the optimal beam in a large narrow-beam codebook. The proposed method can outperform the hierarchical or even the exhaustive search in terms of accuracy and SNR while reducing the beam sweeping overhead by 10×. The probing codebook is learned jointly with a neural network beam predictor and is adapted to capture characteristics of the propagation environment.
​
Machine Learning-Assisted Beam Alignment for mmWave Systems
Sept 2017–May 2019, UT Austin WNCG
Prof. Jeffrey G. Andrews
I proposed a machine-learning (ML) assisted beam training scheme for mmWave initial access (IA) that can accurately select optimal APs and beams without exhaustively searching all APs and beams. The ML algorithm can accurately predict the optimal beam and recommend a set of candidate beams just using GPS feedback from UEs, even with noisy GPS feedback and in a dynamic environment. The proposed method can reduce the search space by approximately 4x for AP selection and over 10x for beam selection while achieving over 95% accuracy.
​
Public Dataset for Network Traffic Classification
Aug 2018–May 2019, UT Austin WNCG
Prof. Jeffrey G. Andrews
I led an undergraduate senior design team to design and implement an automated platform for collecting network traffic data generated by various mobile applications. This project is motivated by the lack of up-to-date network traffic datasets. This dataset can be used to study network traffic classification, malicious attack detection, etc. The dataset is available to the public.
​
Experience-Centric Mobile Video Scheduling Through ML
May 2018–Aug 2018, Samsung Research America
Dr. Vikram Chandrasekhar, Prof. Jeffrey G. Andrews
I proposed ML traffic classification models to enable experience-centric scheduling. The proposed ML algorithms extract simple features from downlink TCP/IP packet headers to classify network traffic and to detect buffer state for video streaming traffic. System-level simulation results show that by differentiating different user services through ML and by modifying a proportional fair (PF) scheduler accordingly, we can reduce buffering time and improve video-user experience without significant affecting conventional FTP users. We obtained 2 patents through this project.